By: Sagar Sidana, Spearheading the Fight Against Climate Change using AI and ML
In this interview, we speak with Mr. Sagar Sidana, an expert in artificial intelligence, machine learning, and data platforms, about the potential applications of these technologies in decarbonization efforts.
Interviewer: Mr. Sidana, thank you for joining us. Let’s start with the fundamentals. How could AI and ML models be adapted or optimized for decarbonization-related tasks?
Sagar: When it comes to AI and ML models in the decarbonization space, there are several potential approaches. Transformer models, which have been successful in natural language processing, could be adapted for time series forecasting in energy demand and renewable energy production.
Reinforcement learning algorithms could potentially be used for optimizing energy systems. These models can learn to make complex decisions in dynamic environments, which could be crucial for managing smart grids with high penetration of renewable energy sources.
Another interesting concept is the use of federated learning in this domain. It could allow for training models on decentralized data, which might be particularly useful when dealing with sensitive energy consumption data from various sources.
Interviewer: Interesting. Could you discuss the potential challenges in data processing and management when it comes to climate-related datasets? How might modern data platforms address these issues?
Sagar: Climate-related datasets present unique challenges due to their scale, complexity, and heterogeneity. We’re often dealing with massive amounts of data from diverse sources – satellite imagery, IoT sensors, weather stations, and more.
Modern data platforms could address these challenges in several ways:
- Scalable Storage and Processing: Cloud-native platforms could handle petabyte-scale climate datasets. These platforms allow for distributed processing, which could be crucial for computationally intensive climate models.
- Data Lakes and Lakehouses: Data lake architectures allow for storage of raw, unstructured data at scale. Some platforms bring ACID transactions to data lakes, which could ensure data integrity in these large, complex datasets.
- Stream Processing: Given the real-time nature of many climate-related data sources, stream processing engines could become increasingly important. They could allow for real-time analysis of sensor data, which might be crucial for applications like early warning systems for extreme weather events.
- Data Governance and Lineage: With the complexity of climate datasets, maintaining data provenance is crucial. Tools for maintaining data lineage could ensure transparency and reproducibility in climate research.
Interviewer: You mentioned IoT sensors. How could edge computing be leveraged in decarbonization efforts, particularly in relation to AI and ML models?
Sagar: Edge computing could play a crucial role in decarbonization efforts, especially in scenarios where real-time decision making is critical. Here are a few potential applications:
- Smart Grids: Edge devices could run lightweight ML models for local load balancing and fault detection. This could reduce the need for constant communication with centralized systems, potentially improving efficiency and resilience.
- Industrial IoT: In manufacturing and industrial settings, edge AI could be used for real-time energy optimization. Models running on edge devices could make instant decisions to adjust processes for optimal energy efficiency.
- Smart Buildings: Edge computing could allow for real-time analysis of building sensor data. ML models running on edge devices could make immediate adjustments to HVAC systems, lighting, and other energy-consuming systems based on occupancy and environmental conditions.
- Renewable Energy: For wind turbines and solar panels, edge computing could enable rapid adjustments based on local conditions. ML models could potentially optimize the angle of solar panels or the pitch of wind turbine blades in real-time.
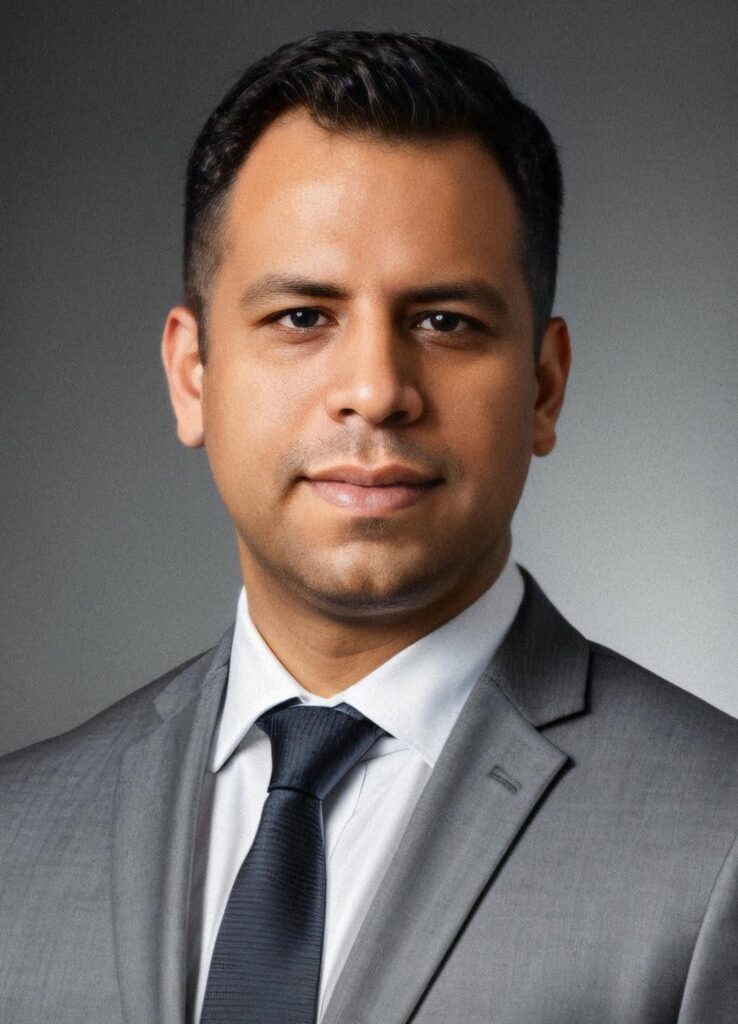
The key advantage of edge AI in these scenarios would be the ability to make instant decisions without the latency of cloud communication, which could be crucial for real-time energy optimization.
Interviewer: Interesting. Now, let’s talk about model interpretability. How could the ‘black box’ problem in AI models be addressed for critical decarbonization applications?
Sagar: Model interpretability is indeed a critical issue, especially when these models could be used to make decisions that have significant environmental and economic impacts. This challenge could be tackled on several fronts:
- Explainable AI (XAI) Techniques: Techniques like SHAP (SHapley Additive exPlanations) and LIME (Local Interpretable Model-agnostic Explanations) could provide post-hoc explanations for complex models like deep neural networks.
- Hybrid Models: There’s potential in hybrid models that combine the power of deep learning with more interpretable models like decision trees. For instance, in energy demand forecasting, a neural network could be used for feature extraction, followed by a decision tree for the final prediction, allowing for better interpretability.
- Attention Mechanisms: In sequence-based models used for time series forecasting, attention mechanisms could not only improve model performance but also provide insights into which parts of the input sequence the model is focusing on for its predictions.
- Causal AI: There’s potential in causal AI methods, which aim to uncover causal relationships rather than just correlations. This could be particularly important in climate science, where understanding causal mechanisms is crucial for developing effective interventions.
- Model Cards and Datasheets: Practices from the responsible AI community, such as creating model cards and datasheets, could be adopted. These could document the intended uses, limitations, and potential biases of models and datasets, promoting transparency and responsible use.
Interviewer: That’s comprehensive. Now, let’s turn our attention to Generative AI. How could this technology be applied in the context of decarbonization efforts?
Sagar: Generative AI is indeed one of the most exciting frontiers in artificial intelligence, and its potential applications in decarbonization are numerous. Here are a few key areas where we could see impactful applications:
- Materials Discovery: Generative models, particularly Generative Adversarial Networks (GANs) and Variational Autoencoders (VAEs), could be used to accelerate the discovery of new materials for clean energy technologies. These models could potentially generate and screen novel molecular structures for more efficient solar cells, better battery electrolytes, and more effective catalysts for carbon capture.
- Synthetic Data Generation: In climate modeling, we often face issues with data scarcity or gaps in historical records. Generative models could create synthetic climate data that maintains the statistical properties of real data. This could be particularly useful for training other ML models or for testing climate scenarios where we lack real-world data.
- Optimizing Energy Systems: Generative models could be used to create and optimize complex energy system designs. For example, generative algorithms could propose novel configurations for renewable energy farms, optimizing for factors like land use, energy output, and grid integration.
- Scenario Generation for Policy Making: Generative models could be employed to create diverse, plausible scenarios for climate change impacts and mitigation strategies. This could help policymakers understand the range of possible outcomes and design more robust policies.
- Generative Design in Architecture: In the built environment, generative design algorithms could create energy-efficient building designs, optimizing for factors like natural lighting, thermal performance, and material use.
Interviewer: Looking ahead, what do you see as the potential future developments in AI, ML, and Generative AI for decarbonization efforts?
Sagar: There are several exciting possibilities on the horizon:
- Multi-modal AI: We could see models that work across different types of data – text, images, numerical data, and even molecular structures. This could lead to more holistic solutions in areas like integrated energy system design.
- AI for Closed-loop Systems: There’s potential for AI models in closed-loop systems, where the AI could propose solutions, test them in simulations, learn from the results, and iterate. This could be transformative for adaptive climate mitigation strategies.
- AI-augmented Scientific Discovery: AI could increasingly augment human scientists in formulating hypotheses and designing experiments, potentially leading to breakthroughs in clean energy technologies.
- Explainable AI: As these models could be used for critical applications, we might see more focus on making them interpretable and explainable, ensuring transparency in decision-making processes.
- AI for Circular Economy Design: We might see more applications in designing products and systems for a circular economy, where AI models could optimize for recyclability and minimal waste throughout the product lifecycle.
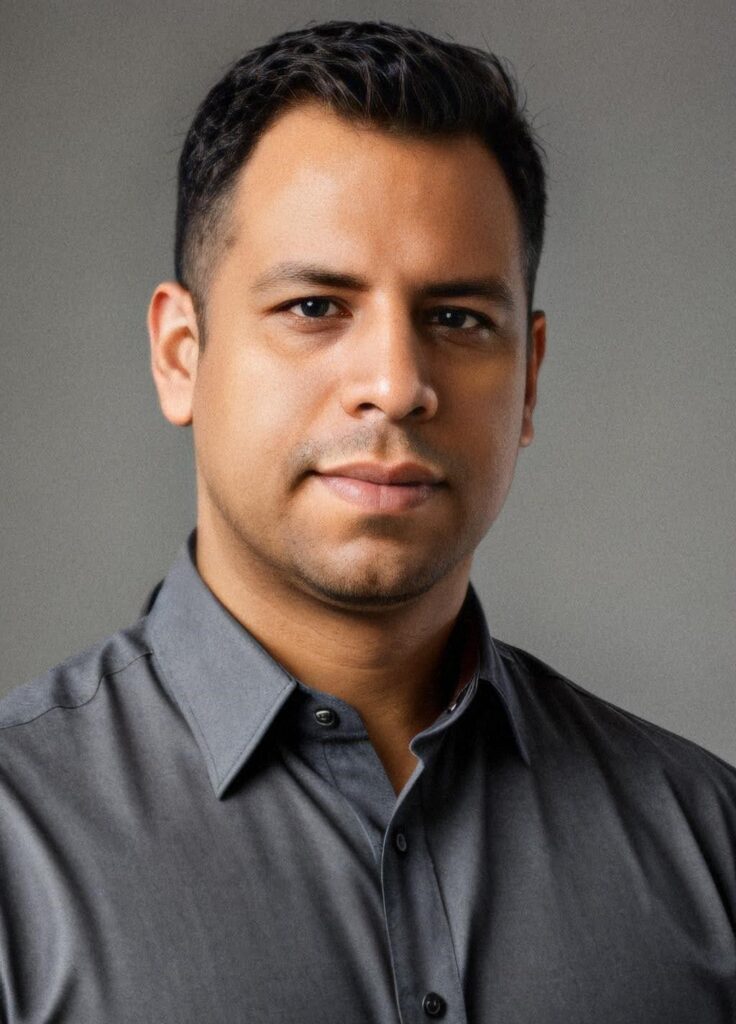
The key will be to develop these technologies responsibly, always keeping in mind their energy consumption and broader environmental impact. The goal should be to ensure that the benefits of using AI in decarbonization efforts significantly outweigh the resources required to develop and deploy these systems.
Interviewer: Thank you, Mr. Sidana, for this comprehensive overview of the potential applications of AI, ML, data platforms, and Generative AI in decarbonization efforts. Your insights into both the current possibilities and future prospects of these technologies are invaluable.
Sagar: It’s been my pleasure. The intersection of these advanced technologies with decarbonization efforts is a field full of potential. While the challenges are significant, I’m optimistic about the possibilities for AI, particularly Generative AI, to contribute to our progress towards a sustainable, low-carbon future. The key is to continue exploring these technologies responsibly, always keeping our ultimate goal of environmental sustainability at the forefront of our efforts.
Published by: Khy Talara