By: Ethan Rogers
From Data To Growth
Artificial Intelligence and data-driven technologies are transforming industries at an unmatched pace. Businesses that tap into AI possess a competitive edge, while those that fail to spot its potential risk lag. Regardless of the widespread availability of AI tools, numerous companies still struggle to integrate them strategically with precision. Being proficient in Data Engineering and MLOps acts as a guide, helping professionals bridge the gap between raw data and intelligent decision-making.
The book(Mastering Data Engineering and MLOps: Building Scalable Pipelines for AI-Driven Decision Making) introduces AI concepts and guides professionals through real-world applications. It dives deep into how structured data engineering and MLOps frameworks empower businesses to transform disintegrated information and actionable insights. By focusing on efficiency, scalability, and automation, the readers get prepared to build AI-powered systems that are innovative but also actionable and sustainable in the long run. Whether you’re an IT leader, an AI practitioner, or a data engineer, this book serves as a blueprint for acquiring data-driven success.
Turning Raw Data Into Business Gold
Data without proper structure remains an untapped goldmine. The unprocessed and raw data holding enormous potential remains chaotic and unusable unless the proper engineering is done. Mastering Data Engineering and MLOps lay out the fundamental principles that help businesses transform disorganized data into meaningful, business-driven insights.
This insightful book covers how data collection, storage, and transformation act as the spine of AI-driven decision-making. It details how modern organizations rationalize data pipelines, ensuring accuracy, consistency, and efficiency. Going deeper into best practices endows readers with the capability to build adaptable systems that are optimized to progressing data needs.
The focus is not just on technical competency ensuring making data operational. Businesses flourish when data is refined and structured, making it possible for them to do data-driven decision-making, trend detection, and streamlined workflows. Data engineering professionals can utilize this book to achieve in-depth knowledge and cultivate proficiency in real-world scenarios.
Bridge The Gap For Success
Building an AI model is only half the journey. The real challenge begins with deploying and maintaining it in real-world scenarios. MLOps emerges as the critical bridge between model development and practical implementation, ensuring that AI systems operate with scalability, efficiency, and continuous improvement.
MLOps brings reliability and structure to the AI model, ensuring seamless integration with existing business processes and preventing business degradation. By automating key tasks, MLOps makes the model more adaptable and resilient in dynamic environments. AI remains a valuable asset for a business that deploys it with precision.
Building Bulletproof Pipelines
An AI system is only as strong as its data pipeline. Without a well-structured pipeline, An AI system centers on the strength of its data pipeline. Missing a well-structured pipeline can cause a business to face significant risks like inefficiency, inaccuracy, and security vulnerability. Organizations can process large volumes of information efficiently with scalable data pipelines
Cloud-based solutions coupled with scalable data pipelines and effective security measures are fundamental for ensuring data integrity, data processing, and resulting success.
Level Up To Ai Toolkit
AI-driven solutions depend on a powerful ecosystem of robust tools. Apache Spark facilitates distributed data processing along with massive datasets. Kubernetes managers containerized applications securing smooth AI model deployment across diverse environments.
On the other hand, AI is simplified across multiple platforms by simplifying the packaging and portability offered by Docker. For automating updates and system reliability enhancement, CI/CD pipelines streamline development workflows
CI/CD pipelines work in a way that streamlines development workflows. This specific task is done by automating reliability and system reliability enhancement. To make oneself a professional, these tools need to be mastered resulting in building scalable and robust AI-driven infrastructures.
Ai Lifecycle From Development To Deployment
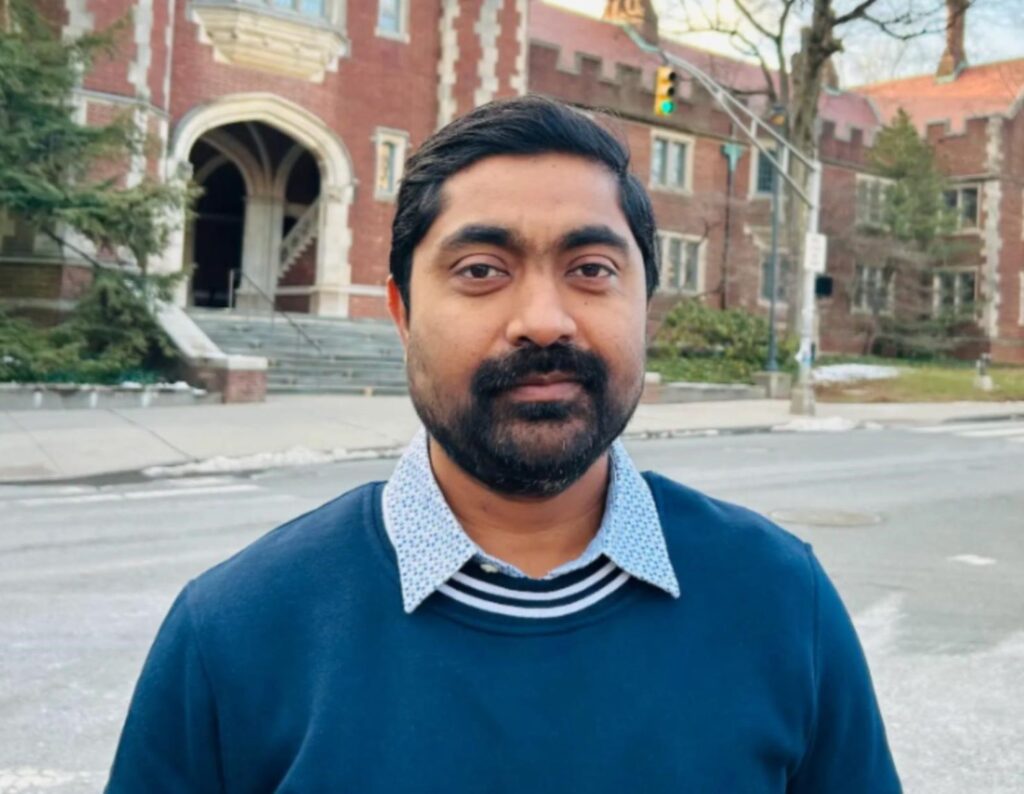
The first baby step is just to deploy AI models, the real challenge is ensuring they remain efficient over time. Data inconsistencies, model drift, and performance degradation can result in a reduction of an AI system’s effectiveness. Such risks can be mitigated by continuous monitoring resulting in models to be accurate and responsive to data patterns.
Refining data pipelines and optimizing computational resources are a few effective troubleshooting techniques that help to maintain system reliability. Ethical AI usage and maintaining compliance with industry regulations are ensured by data governance. Prioritizing AI maintenance maximizes the return on AI investments and enhances longevity.
AI in Action In The Real World
AI is no longer a futuristic thing, it has already transformed organizations and businesses. The industries leveraging data engineering and AI to optimize processes have enhanced customer experiences. Success stories from different sectors reveal how AI frameworks are reducing operational costs, increasing efficiency, and improving decision-making.
These are not just cases, they demonstrate how AI can impact when integrated properly into operations
Why This Book Is A Must-Read For AI and Data Professionals
AI-influenced data-driven strategies are proving to be the backbone of modern organizations inevitable for professionals to stay ahead. Mastering Data Engineering and MLOps: Building Scalable Pipelines for AI-Driven Decision-Making equips the readers with applicable skills for building scalable AI systems.
By filling the gap between theory and application, this book develops the sense of winning real-world challenges in AI implementations. Whether it is optimizing workflows, deploying AI models, or even managing data pipelines, the knowledge acquired here makes professionals ready to drive innovation in the fast-paced, evolving digital landscape.
Meet The Trailblazer: Visionary Mind Behind AI and Data Engineering
Over 17 years of experience made Jyotipriya Das a recognized leader in AI-driven healthcare innovations. His profound expertise in cutting-edge Artificial Intelligence, Machine Learning, and Medical Imaging has garnered him international acclaim.
The contributions of Jyotipriya feature high-impact research, AI solutions, and the astonishing development of data curation pipelines that boost AI model efficiency and accuracy.
Jyotipriya’s unparalleled achievements have placed him at the supreme of his field. The innovations are being widely adopted in the healthcare sector. His accomplishments made him receive both national and international acclaim, consolidating his reputation as a pioneer in the development of AI-driven medical imaging technologies. Through his technical advancements and research, Jyotipriya continues to push the boundaries of AI applications, leaving a significant impact on the entire industry.
Published by Tom W.